Artificial Intelligence & Machine Learning / Enterprise AI implementation
Weekly Artificial Intelligence & Machine Learning / Enterprise AI implementation Insights
Stay ahead with our expertly curated weekly insights on the latest trends, developments, and news in Artificial Intelligence & Machine Learning - Enterprise AI implementation.
Recent Articles
Sort Options:
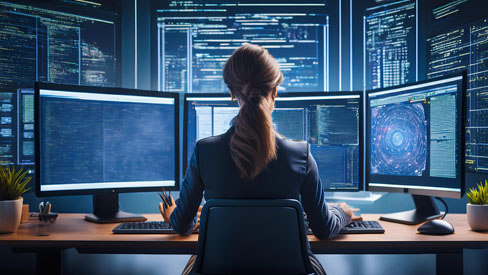
Fini
The article explores the effectiveness of enterprise-grade AI support, highlighting its practical applications and benefits for businesses. It emphasizes the importance of reliable AI solutions in enhancing operational efficiency and driving innovation in various industries.

We Built The AI Model. Now What?
A recent article reveals that many executives mistakenly think building AI models is the toughest challenge in enterprise AI. Instead, the complexities of implementation and integration pose greater hurdles for organizations looking to leverage artificial intelligence effectively.

The Three Foundational Pillars Of Every Enterprise AI Strategy
Accelerated advancements in Artificial Intelligence (AI) and Large Language Models (LLMs) are transforming industries and enterprise strategies, highlighting the significant impact of these technologies on the future of business and innovation.
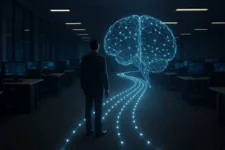
How to Engineer AI Adoption and Value Across the Enterprise
AI development is at a crucial juncture, where fostering trust and demonstrating ROI are essential for enterprise adoption. Organizations that strategically integrate AI into operations while ensuring accessibility and efficiency will unlock its transformative potential and drive significant business value.
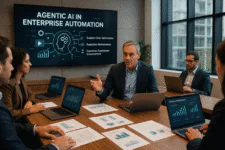
Evaluating Where to Implement Agentic AI in Your Business
Agentic AI is poised to transform industries by enabling autonomous decision-making and real-time adaptability. Businesses must strategically assess integration opportunities to enhance efficiency while balancing automation with human oversight for optimal outcomes.

Enterprise Intelligence: Why AI Data Strategy Is A New Advantage
The future of enterprise AI hinges not just on computing power, but on organizations innovating data movement in real time. This shift promises to redefine industry standards and enhance operational efficiency, according to industry experts.
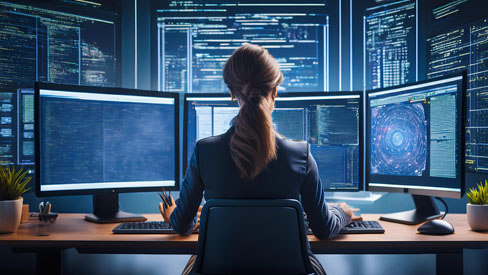
How AI Agents Are Transforming Enterprise Automation Architecture
The article explores the evolution of intelligent autonomous systems, highlighting the impact of machine learning and natural language processing. It offers an exclusive guide on AI agents for enterprise automation and their transformative effects across multiple industries.
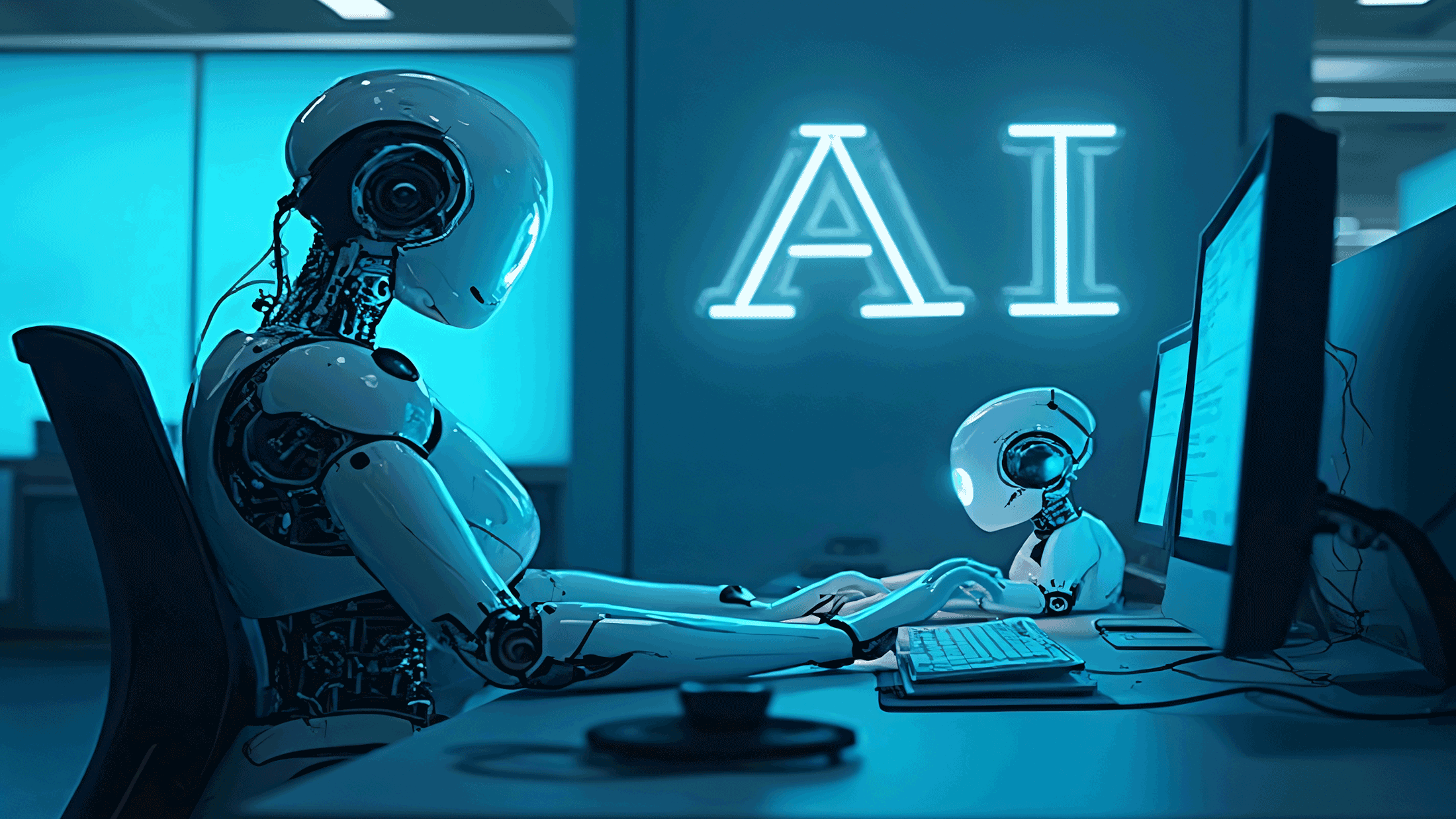
Why agent systems are key to unlocking enterprise AI in the UK
Organizations are increasingly shifting towards AI agent systems to overcome challenges in scaling Generative AI projects. These systems integrate specialized components for tailored solutions, enhancing productivity and trust while addressing data governance and security concerns in the evolving AI landscape.

Mistral comes out swinging for enterprise AI customers with new Le Chat Enterprise, Medium 3 model
Mistral AI is actively working to enhance scalable, privacy-respecting AI adoption for contemporary enterprises, aiming to simplify integration and promote responsible use of artificial intelligence in business environments.
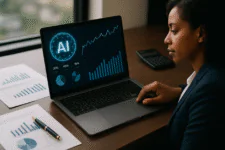
Utilizing AI for Better Business Insights: Minimize Costs, Maximize Results
AI is revolutionizing business intelligence by automating data preparation and enhancing decision-making. Companies like GE Aerospace leverage AI for predictive analytics and personalized insights, while emerging trends like synthetic data and explainable AI promise to further improve operational efficiency and strategic growth.

Scaling AI Agents In The Enterprise: Frameworks, Processes And Best Practices
Organizations can effectively integrate AI into daily operations by implementing a well-planned strategy, moving past experimental use cases to enhance efficiency and innovation in their workflows, according to insights from industry experts.
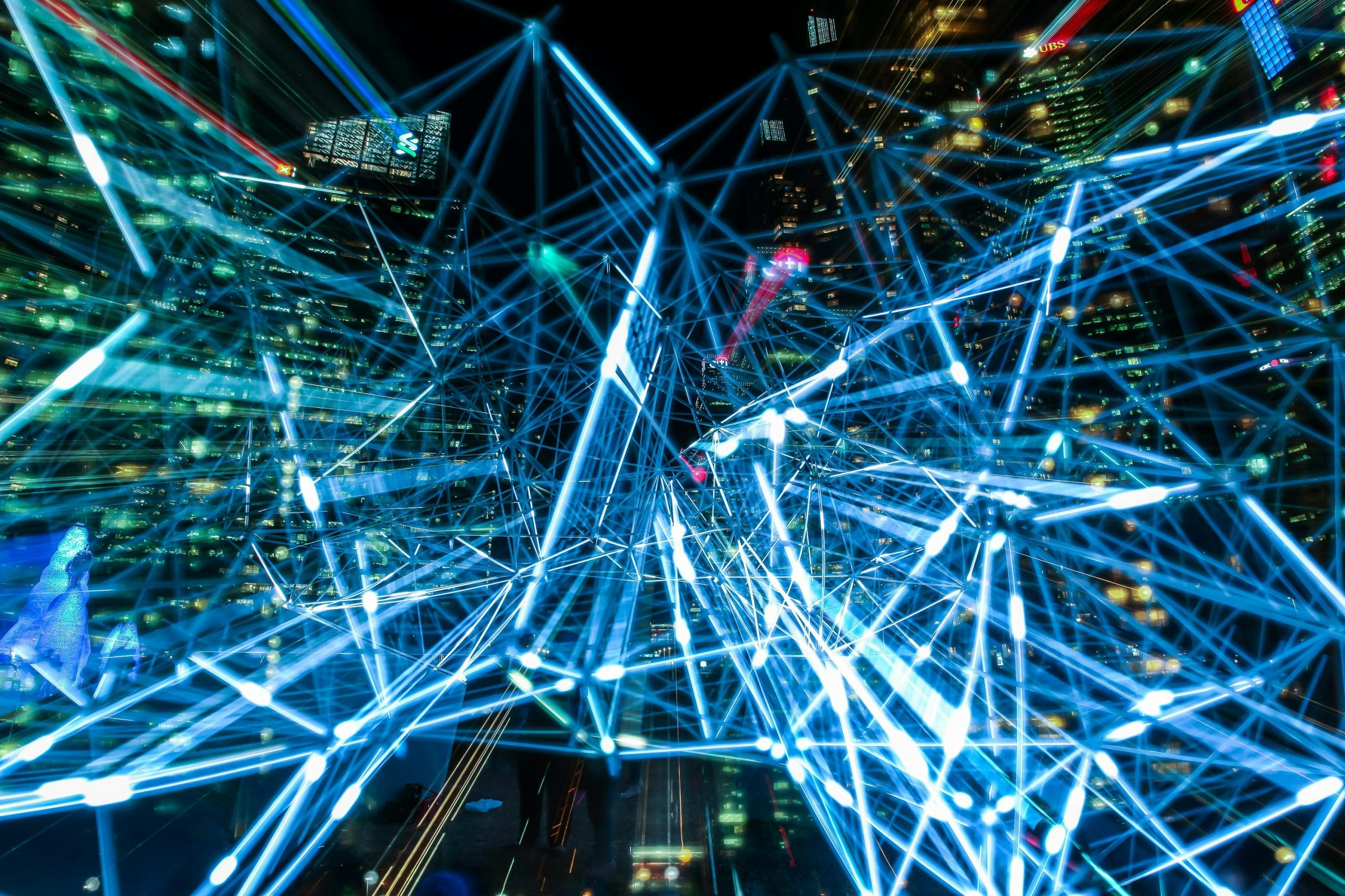
Enterprise AI Is Evolving — From Innovation to Execution
AI innovation is rapidly advancing, with new generative models and breakthroughs emerging weekly. However, the real challenge for enterprises lies in effective execution, emphasizing the need for comprehensive systems over isolated tools to harness this potential.